Building a Customer Database for Financial Analysts
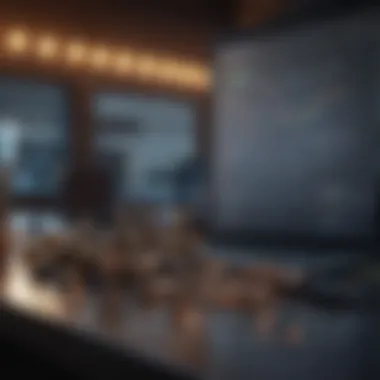
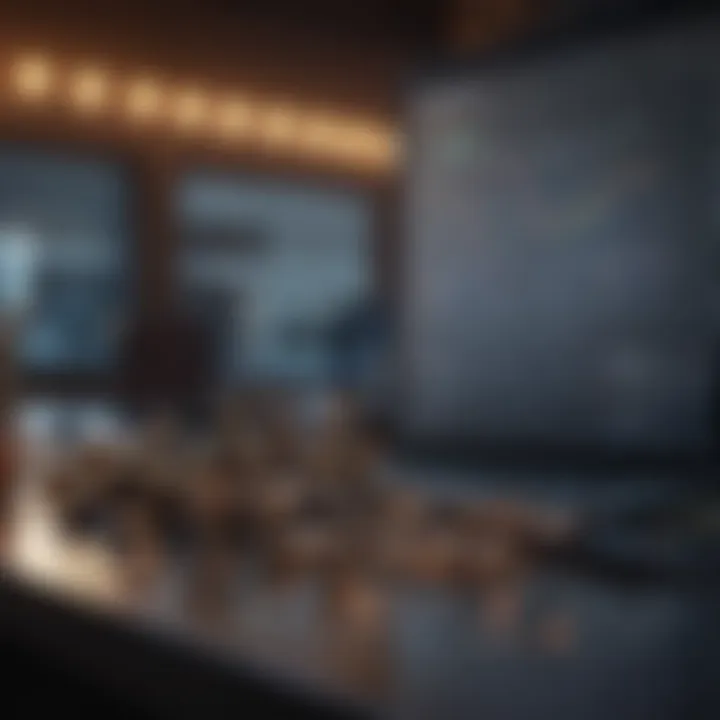
Intro
Creating a customer database is a fundamental task for financial analysts and services firms. A well-structured database allows analysts to gain insights into customer behavior and preferences, essential for making informed investment decisions and improving service delivery.
This guide provides an in-depth understanding of how to create a customer database specifically for financial services. It focuses on the process, the tools required, and emphasizes the importance of data privacy, analytical best practices, and leveraging technology for effective data management.
Market Overview
A customer database within the context of finance intersects with ongoing market trends, economic indicators, and global influences.
Current Market Trends
The financial landscape has experienced significant fluctuations in recent years. Staying abreast of current trends, such as the rise in retail trading and the increasing adoption of digital currencies, is crucial. Analysts should note how these trends impact customer investment behavior, leading to the necessity for tailored database solutions.
Economic Indicators Impacting the Market
Economic indicators, including GDP growth rates, unemployment figures, and consumer confidence indexes, directly affect investment patterns. Analysts must analyze how these indicators interact with customer data to recognize emerging patterns and adapt services accordingly.
Global Influences and Major Events
Global events, such as geopolitical tensions, trade agreements, and pandemics, can have drastic effects on the market. Understanding how external factors influence customer behavior is vital for developing a responsive database.
Sector Analysis
When creating a comprehensive customer database, sector analysis becomes an important component. It allows analysts to understand sector-specific performance and investment opportunities.
Performance of Major Sectors
Different sectors demonstrate unique performance metrics. For instance, technology stocks may show high growth potential, while traditional industries may exhibit stability. Understanding these dynamics assists analysts in crafting targeted strategies tailored to customer needs.
Comparative Analysis Between Sectors
A comparative approach provides insight into which sectors outperform others over time. By including this analysis in the database, analysts can guide clients better and recommend informed investment strategies based on sector performance.
Emerging Sectors to Watch
Identifying emerging sectors can give clients an edge in their investment decisions. Sectors such as renewable energy or biotechnology present growing opportunities. Tracking customer interests in these areas through the database can support recommendation efforts.
Stock Recommendations
An effective customer database can facilitate stock recommendations tailored to individual investors.
Featured Stocks of the Month
Highlighting top investments monthly encourages clients to engage with the market. These selections should be based on solid research and data analysis to ground recommendations in factual information.
Long-term vs.
Short-term Picks
Different investors have varying objectives. A database can categorize stocks into long-term and short-term holdings, guiding clients based on their specific goals and risk tolerance.
Criteria for Stock Selection Guidelines
Developing clear guidelines for stock selection helps maintain a consistent approach when making recommendations. Criteria may include financial health, market trends, and customer preferences.
Investment Strategies
Incorporating robust investment strategies into the customer database significantly enhances its value.
Fundamental vs.
Technical Analysis
Distinguishing between fundamental and technical analysis will help analysts know which methodologies suit their clients best. Data within the database can indicate the most effective strategies for different customer profiles.
Risk Assessment and Management Strategies
Understanding risk is crucial in finance. A database should contain risk profiles for customers, aiding analysts in creating personalized investment strategies that align with risk appetites.
Asset Allocation Tips
Effective asset allocation is essential for effective risk management. Incorporating asset allocation tips tailored to individual investor profiles will enhance the utility of the database.
Educational Resources
Providing educational resources through the customer database can empower clients, fostering informed investment choices.
Glossary of Stock Market Terms
A glossary can demystify complex terminology, making the information more accessible. This resource supports users in understanding the language of finance.
Tutorials on Investment Basics
Offering tutorials cater to novice investors, helping them grasp the foundational concepts of investing while engaging more actively with the market.
Webinars and Interactive Tools
Facilitating learning through webinars and interactive tools allows clients to deepen their knowledge and gain insights leveragable in real-time.
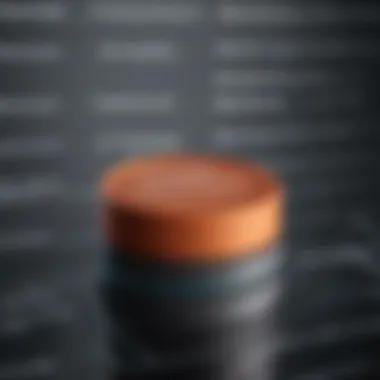
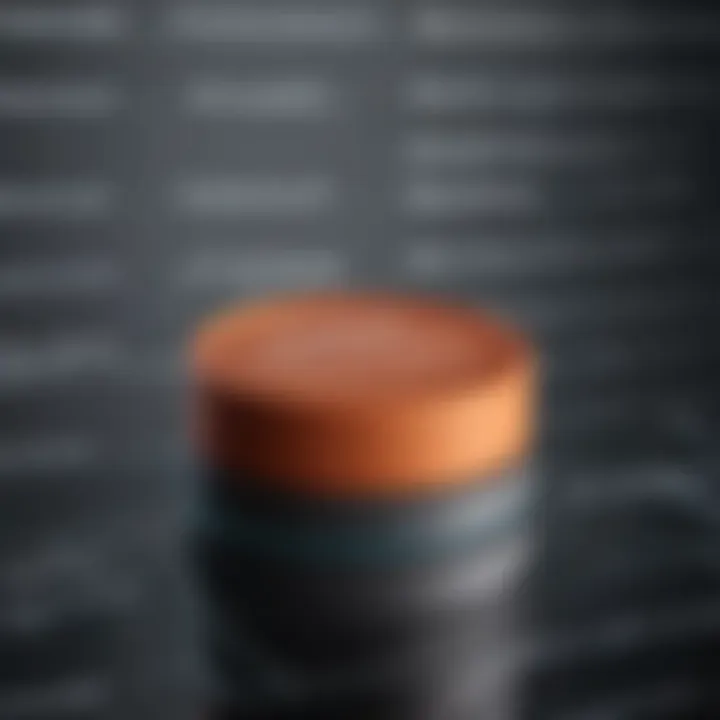
A customer database should be a dynamic entity, adjusting to evolving market conditions, customer preferences, and emerging trends.
Preamble to Customer Databases
Customer databases have become essential tools in the financial sector. They allow analysts to collect and manage vast amounts of information about clients. This greatly aids in tailoring services to meet individual needs. Furthermore, an efficient database supports better strategic decisions by analyzing customer behavior and preferences.
In an era where data drives decisions, understanding customer databases is critical. These systems not only store data but also enable financial analysts to derive actionable insights. By having access to segmented customer information, professionals can gauge market trends, enhance customer engagement, and bolster investment strategies. Companies that prioritize customer database management often see improved service delivery and higher client satisfaction.
Definition and Purpose
A customer database is a centralized repository that stores information about customers, including personal details, transactions, and interactions. Its primary purpose is to organize this information in a way that enables easy access and analysis. In finance, where customer relationships are essential, a well-structured database allows for a nuanced understanding of client behavior.
Key Functions include:
- Data Storage: Securely collects personal and financial data.
- Data Analysis: Facilitates the analysis of customer patterns and trends.
- Customer Relationship Management: Enhances relationships through tailored communication and services.
By integrating all relevant data, the database acts as a powerful tool for financial analysts, fostering informed decision-making and strategic planning.
Importance in Financial Services
The role of customer databases in financial services cannot be overstated. They are integral in creating a more personalized experience for clients. Analyzing data allows professionals to:
- Make informed investment choices.
- Identify new market opportunities.
- Anticipate customer needs based on historical data.
"Data is the new oil." This statement highlights how valuable well-managed data is in driving financial success.
Identifying Data Requirements
Identifying the specific data requirements is crucial for constructing an effective customer database. This step sets the foundation for understanding customer profiles, behaviors, and preferences. By pinpointing what data to collect, financial analysts can ensure they gather relevant and insightful information, which in turn leads to improved decision-making processes. Moreover, focusing on precise data elements minimizes the risk of information overload, allowing for more efficient data management.
Types of Customer Data
Understanding the various types of customer data is essential. Each type brings unique advantages that contribute to enhanced strategies in the financial sector. The three primary types include Personal Information, Transactional Data, and Behavioral Insights.
Personal Information
Personal Information encompasses basic details about customers, such as name, address, contact numbers, and date of birth. This data serves as the foundation for engaging with customers on a personal level. By having accurate personal information, financial analysts can tailor their communication and marketing strategies effectively.
- Key Characteristic: One significant trait of personal information is its ability to create individual customer profiles. This is beneficial in customizing services for specific segments of clients.
- Unique Feature: The unique aspect of personal information is its inherent stability. Once collected, it does not change frequently, making it a reliable cornerstone for building customer relationships.
- Advantages/Disadvantages: While personal information is incredibly valuable, it also requires stringent security measures. Failing to protect this data can lead to significant legal repercussions and loss of customer trust.
Transactional Data
Transactional Data refers to details about customers' interactions with financial services. This includes purchase history, frequency of transactions, and payment methods. This type of data is crucial in understanding how customers engage with financial products.
- Key Characteristic: The most prominent feature of transactional data is its ability to reflect customer behavior in real-time. This insight is vital for analyzing trends and adjusting services accordingly.
- Unique Feature: Transactional data often allows for a direct correlation with spending habits, aiding in identifying high-value customers or potential risk factors.
- Advantages/Disadvantages: The main advantage is its dynamism; it updates automatically with each transaction. However, the potential challenge lies in managing the system to capture accurate and timely updates.
Behavioral Insights
Behavioral Insights delve into customers' patterns, preferences, and choices. This includes engagement metrics, responses to marketing stimuli, and overall satisfaction levels. Understanding these factors is fundamental for creating targeted initiatives that resonate with specific customer segments.
- Key Characteristic: One important feature of behavioral insights is the predictive capability. These insights can forecast future customer actions, allowing analysts to strategize proactively.
- Unique Feature: Behavioral data is often gathered through engagement analytics, making it a dynamic source of information that reflects evolving customer interests.
- Advantages/Disadvantages: Insights derived from this data can enhance engagement and retention strategies. On the downside, the interpretation can be complex, often requiring advanced analytical tools and expertise.
Data Quality Standards
Ensuring high data quality is paramount for any customer database. It is essential to have standards that guide data collection, entry, and management practices. High-quality data leads to more accurate analyses and better-informed strategic decisions.
- Key Standards: Consistency, accuracy, and completeness are core standards that need to be upheld throughout the data lifecycle.
- Importance: Poor quality data can lead not only to flawed analyses but also to misdirected marketing efforts and lost opportunities.
Balancing these aspects while identifying data requirements will enrich the customer database, providing a robust platform for financial analysts. By focusing on quality and relevance, insights derived from this data can significantly influence financial strategies and operational effectiveness.
Selecting Database Technology
Selecting the right database technology is crucial for effective customer data management in the financial sector. The choice of database impacts data retrieval, user experience, and overall analysis capabilities. Financial analysts must consider various factors, including scalability, data structure, and the type of queries they anticipate running. A well-chosen database technology can enhance insights into customer behavior, streamline operations, and improve decision-making.
Types of Databases
Relational Databases
Relational databases are widely used in financial services for their structured data management. They rely on a table-based architecture where data is organized into rows and columns.
A key characteristic of relational databases is their use of SQL (Structured Query Language) to enable complex queries. This is beneficial for analysts who need to generate detailed reports and perform intricate data analysis.
One unique feature is their ability to enforce data integrity through constraints. This means analysts can be more confident that the data is accurate and consistent. However, a disadvantage is that they can struggle with very large datasets and may require significant tuning for optimal performance.
NoSQL Solutions
NoSQL databases offer a flexible approach to data storage, supporting unstructured and semi-structured data. This is especially advantageous in financial services where customer behavior data can vary widely.
A key characteristic of NoSQL solutions is their scalability, which allows them to handle vast amounts of data with ease. They are designed for quick read and write access, making them suitable for real-time data analytics.
A notable feature of NoSQL databases is their schema-less nature, enabling analysts to store data without rigid predefined structures. On the other hand, they may lack some of the robust transactional capabilities found in relational databases, making them less ideal for certain financial operations.
Cloud-Based Options
Cloud-based databases provide a scalable and flexible solution for financial analysts. These databases leverage cloud computing resources, enabling organizations to access and manage data remotely.
One key characteristic of cloud-based options is their inherent scalability, allowing businesses to adjust resources as needed. This flexibility supports growing data demands without the need for extensive hardware investments.
A unique aspect of cloud databases is their potential for automatic updates and maintenance, freeing analysts from routine tasks. However, this cloud dependence can raise concerns about data security and compliance, particularly in regulated financial environments.
Evaluating Database Platforms
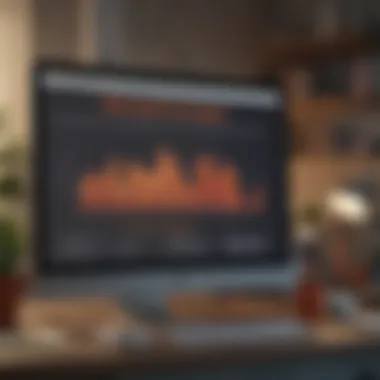
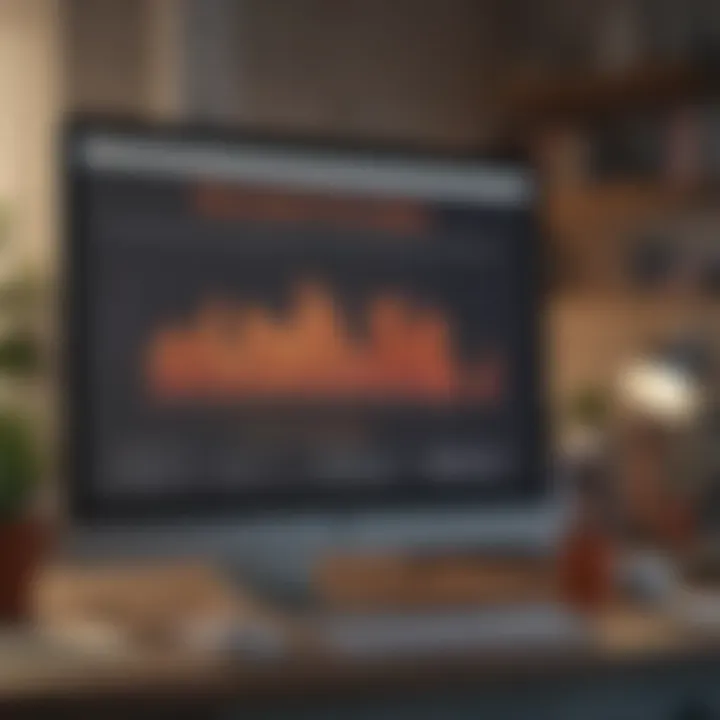
When evaluating database platforms, analysts must consider factors such as performance, cost, and ease of integration with existing systems. A comprehensive assessment helps ensure the selected technology aligns with the organization’s data strategy and future goals. Key considerations might include:
- Performance metrics: Speed and efficiency of querying.
- Cost-effectiveness: License fees versus potential ROI.
- Support and documentation: Access to resources for troubleshooting.
- Integration capabilities: Compatibility with other tools and software.
The right database technology not only influences current operations but also shapes future data management strategies.
Data Collection Methods
Data collection methods are central to building an effective customer database. They define how data is acquired, ensuring that the information collected is accurate, relevant, and valuable for analysis in financial services. The benefits of effective data collection can be substantial. It helps in understanding customer preferences and behaviors, allowing analysts to structure more personalized financial products and services. Moreover, it allows institutions to remain competitive in an evolving market by staying attuned to client needs and trends.
Surveys and Feedback Forms
Surveys and feedback forms serve as primary tools for gathering data directly from customers. They can be highly beneficial for financial analysts aiming to enhance their understanding of client satisfaction and expectations. These tools can cover various aspects, such as customer experience with services or products, pricing feedback, and overall service delivery:
- Target Specific Groups: Surveys can be designed to target specific customer segments relevant to financial products.
- Flexible Formats: They offer multiple formats, including online forms, telephone interviews, or in-person surveys, allowing for broader reach.
- Quantitative and Qualitative Data: Surveys can collect both quantifiable data, which can be analyzed statistically, and qualitative feedback that provides deeper context.
Constantly updating these surveys ensures that the data remains current, reflecting changes in customer sentiment over time. However, it is essential to consider survey fatigue and ensure that questions are concise. Incentivizing participation could also enhance response rates.
Integrating with Existing Systems
Integration with existing systems is crucial for seamless data collection. Financial analysts must ensure that new data collection methods can work in synergy with current databases and systems. This integration helps in minimizing redundancy and ensuring that the data flow is consistent across platforms:
- Streamlined Processes: Systems like CRM software or investment management platforms should be able to incorporate survey results and feedback instantly.
- Data Accuracy: Integration aids in maintaining data integrity, as new customer insights can update existing customer profiles without manual entry.
- Enhanced Analysis: When data is centralized, financial analysts can perform more comprehensive data analyses, leading to better insights regarding customer behavior and market trends.
Exploring API connections and middleware solutions can facilitate this integration effectively, ensuring the data collection framework aligns with the organizational data strategy.
Data Privacy and Compliance
Data privacy and compliance are critical components in the process of creating a customer database, especially in the financial sector. With the increasing scrutiny around data handling practices, organizations must ensure that they not only collect but also protect customer information adequately. Regulatory frameworks guide financial institutions in maintaining compliance, but there are also best practices for data security that enhance overall trust and credibility in operations.
Regulatory Frameworks
General Data Protection Regulation
The General Data Protection Regulation (GDPR) represents a comprehensive legal framework governing data protection in the European Union. Its significance lies in its focus on individual rights and data privacy, which contributes directly to building more responsible data management practices in organizations. One key characteristic of GDPR is the emphasis on consent; organizations must obtain explicit permission from users before collecting their data. This approach fosters a culture of transparency and accountability.
A unique feature of GDPR is the right to erasure, often referred to as the
Implementing the Database
Implementing a customer database is a crucial step for financial analysts aiming to optimize their data management strategies. At this stage, financial professionals begin to see their planning, research, and analysis come to fruition. The successful implementation of a customer database leads to improved access to vital information and enhanced decision-making capabilities.
Data Entry and Management
Data entry and management involve several critical components that ascertain the integrity and usability of the customer database. Proper data entry ensures every piece of relevant customer information is accurately recorded. This might include personal details, transaction history, and interactions with financial services.
Maintaining a clean database is essential to avoid any errors that could lead to misinformed business decisions. Here are some best practices for data entry:
- Standardization: Set formats for how data is input to ensure consistency.
- Validation Checks: Apply automated checks to confirm the accuracy of the entered data.
- Training: Ensure employees handling data entry are well-trained and familiar with the database tools.
Effective management of the data includes regular updates and maintenance to keep information current. Different software tools can assist in this process. Examples include customer relationship management tools like Salesforce or database management systems like MySQL. Regular oversight ensures that any duplicate or outdated entries are corrected or removed. This can drastically improve the quality of insights drawn from the database.
User Access and Roles
Determining user access and roles within the customer database is another vital aspect to consider. It's important to establish who can access and manipulate data. Not everyone within an organization needs the same level of access. Therefore, implementing a role-based access control (RBAC) system is advisable. This not only helps to secure sensitive data but also simplifies the management of user permissions.
Here are key considerations regarding user access:
- Define Roles: Clearly outline the different roles in your organization and what functionalities each role should have.
- Regular Reviews: Conduct periodic reviews of user access to ensure compliance and relevance to staff functions.
- Utilize Audits: Implement audit trails to monitor who accesses the database and when, enhancing accountability.
By focusing on these elements, financial professionals can ensure that their customer databases are well-implemented, providing a strong foundation for data-driven decisions and strategic planning. Utilizing these methods will empower financial analysts to derive meaningful insights and elevate their business operations.
Maintaining the Customer Database
Maintaining a customer database is a critical aspect that directly influences the effectiveness of financial analysis and customer relationship management. A well-maintained database ensures that the information is accurate, available, and relevant for making informed financial decisions. Financial analysts rely on this data to understand customer behaviour, tailor services, and enhance investment strategies. By focusing on the maintenance of customer databases, financial organizations can derive substantial benefits that contribute to their long-term success.
Regular Data Audits
Regular data audits are essential in the process of maintaining a customer database. These audits help identify inaccuracies, redundancies, and outdated information that may exist within the system. Financial analysts need to develop a structured approach to conducting these audits. This includes setting specific intervals for auditing data, which can be quarterly, semi-annually, or annually depending on the volume of data and business needs.
To conduct effective data audits, organizations can follow these steps:
- Data Validation: Verify the accuracy of the existing data by cross-checking against reliable sources.
- Remove Duplicates: Eliminate duplicate entries in the database to improve efficiency.
- Identify Missing Information: Spot and fill any gaps in the customer data that could affect analytics.
- Evaluate Data Usage: Assess which data points are being used and which are not, allowing for strategic updates to data collection methods.
A systematic approach to data audits allows for better decision-making and enhances the overall integrity of the customer database.
Updating Customer Information
Updating customer information is another crucial element in maintaining a current and useful customer database. As customers evolve, their personal and financial information may change. This might include changes in contact details, preferences, or even their financial status. Regularly updating this information ensures that financial analysts have access to the most pertinent data.
To facilitate effective updates, here are some best practices:
- Establish a Regular Update Schedule: Set a routine for updating customer information. This can include monthly or bi-monthly checks, particularly for high-value clients.
- Encourage Customer Engagement: Actively solicit updates from customers through surveys or during regular interactions, such as when they log into accounts or during service engagements.
- Implement Automated Systems: Use software tools that can automate the process of data collection and updates to minimize manual errors.
Regular updates are not only beneficial for data quality but also improve customer satisfaction by ensuring that communications and offers are relevant and timely.
Maintaining an accurate customer database is a continuous process that requires attention, strategy, and commitment. It forms the backbone of successful financial analysis and decision-making.
Leveraging Data for Insights
Leveraging data effectively is a critical factor in transforming customer databases into powerful tools for financial analysts. The integration of insights derived from customer data not only helps firms to understand their clientele better, but it also enables more informed decision-making processes. By parsing through available information, analysts can identify trends, preferences, and potential areas for growth. This section explores two significant approaches: customer segmentation and predictive analytics.
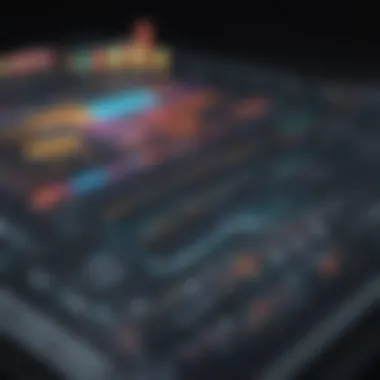
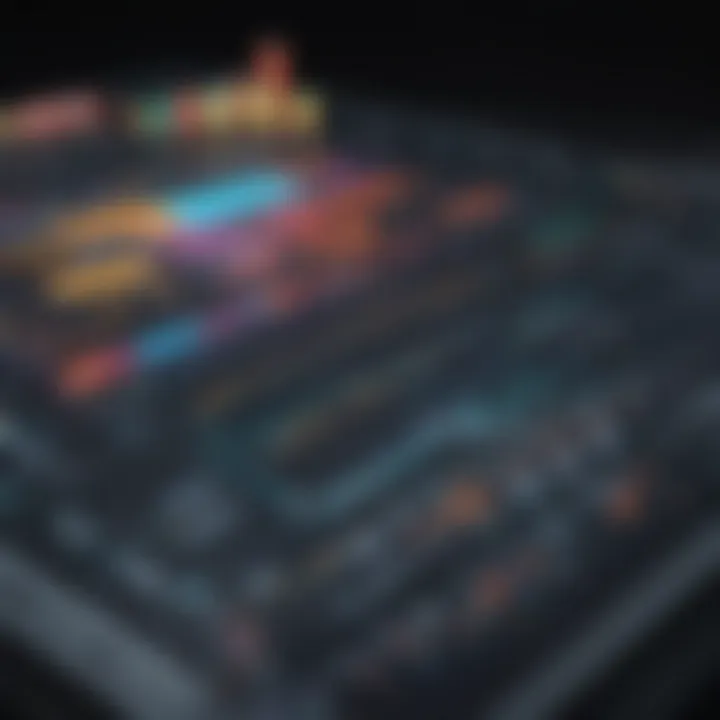
Customer Segmentation
Customer segmentation is the practice of dividing a customer base into distinct groups based on specific criteria. These can include demographics, purchasing behavior, and financial needs. The importance of segmentation lies in its ability to tailor marketing and service delivery effectively.
Benefits of Customer Segmentation:
- Personalization: Allows companies to create tailored messages that resonate with specific customer groups.
- Resource Allocation: Helps allocate resources more effectively, directing marketing efforts to high-value segments.
- Increased Retention Rates: By understanding customer needs better, companies can enhance customer satisfaction and loyalty.
Moreover, segmentation supports targeted product development. For example, an investment firm may create specialized portfolios aimed at different investor profiles, such as conservative, balanced, or aggressive. This strategy aligns services closely with customer expectations.
Predictive Analytics in Financial Services
Predictive analytics employs statistical algorithms and machine learning techniques to analyze historical data and make predictions about future outcomes. In financial services, predictive analytics plays a pivotal role in risk management and investment strategies.
Considerations for Implementing Predictive Analytics:
- Data Quality: The accuracy of predictions is contingent upon the quality of data collected. Therefore, maintaining high standards for data accuracy is fundamental.
- Model Selection: Choosing the right predictive models can impact the reliability of insights. Analysts must understand and test various algorithms to find the best fit.
- Continuous Learning: The models should evolve with new data inputs. This adaptability ensures ongoing accuracy in predictions.
Through predictive analytics, financial analysts can anticipate market movements, optimize portfolio management, and identify potential investment opportunities. This proactive approach fosters smarter decision-making and helps mitigate risks significantly.
Investing based on deep, data-driven insights can lead to sustained growth and profitability.
In summary, leveraging data for insights is essential for the financial sector. Customer segmentation allows for personalized strategies, while predictive analytics equips analysts with tools for future planning. The integration of these elements into customer database management is vital for achieving competitive advantage.
Case Studies and Examples
Case studies offer real-world evidence about the effective implementation of customer databases in financial services. Their value lies in the ability to provide tangible examples of how organizations have navigated the complexities of data management, revealing both the benefits and challenges involved. This section is fundamentally about learning from the experiences of others. By analyzing successful implementations and examining failures, financial analysts can understand best practices and pitfalls, thus enhancing their approach to customer data management.
Successful Customer Database Implementations
In the realm of financial services, several institutions have successfully established robust customer databases. A notable example is JPMorgan Chase. This bank has integrated data from multiple channels, such as online banking, credit cards, and investment services. This integration allows for a comprehensive view of customer behavior, enabling the bank to tailor its services more effectively. As a result, it saw a significant increase in customer satisfaction and retention.
The ability to provide personalized recommendations not only improved users’ experiences but also fostered stronger client relationships. Furthermore, their data-driven approach has enhanced risk management and compliance efforts, reflecting the implementation's multifaceted benefits. Successful case studies exemplify that a well-structured database is not an end but a means to deliver value through insightful data analytics.
Examples also exist in smaller financial tech firms. One such firm, Wealthfront, uses advanced algorithms to analyze customer data. With this, they provide insights that lead to more informed investment decisions for clients. By focusing on automated financial planning and maintaining a user-friendly experience, they have attracted a significant customer base, benefiting from the personalized service they can provide through their effective customer database.
Lessons Learned from Failed Initiatives
On the other side, it is essential to shed light on initiatives that did not achieve the desired outcomes. These cases serve as valuable learning resources. A prominent example is the implementation struggles faced by the Royal Bank of Scotland during the launch of their customer database system. The bank aimed to consolidate data across various products and channels but encountered significant challenges. They faced data integrity issues and employee resistance to new technologies. As a result, the project was delayed, leading to losses in customer trust and operational efficiency.
The key takeaway from this is the importance of change management in any technology implementation. Successful adoption of a customer database requires thorough training for employees, clear communication, and effective project management. Moreover, ensuring data quality right from the start is crucial; otherwise, it leads to issues that can cripple operations down the line.
Lessons from these failed initiatives underline the need for proper planning, training, and maintenance. Failure to address these aspects can turn promising projects into burdens.
Future Trends in Customer Data Management
The landscape of customer data management is evolving rapidly, influenced by advancements in technology and changing market demands. Understanding these trends is crucial for financial analysts aiming to harness customer data effectively. With the ongoing digital transformation, organizations are increasingly focusing on how to leverage these shifts in their data strategies.
Artificial Intelligence and Machine Learning
Artificial Intelligence (AI) and Machine Learning (ML) are profound elements altering the way organizations manage customer data. These technologies are essential in predicting customer behavior and enhancing decision-making processes. Financial analysts can utilize AI models to analyze vast amounts of data quickly. This leads to better segmentation, personalized marketing strategies, and efficient resource allocation.
Key benefits of AI and ML in data management include:
- Enhanced Predictive Analysis: Help in forecasting customer trends based on historical data.
- Automation: Streamlines data processing and reduces human error.
- Improved Customer Insights: Generates deeper understanding of customer preferences and behaviors, contributing to tailored financial advice.
Implementing AI-driven tools can lead to significant insights, which can improve customer satisfaction and retention rates significantly.
Integration with Blockchain Technology
Blockchain technology represents another significant trend in customer data management. This decentralized ledger system enhances data security and transparency. In financial services, where data integrity is paramount, blockchain can streamline transactions and provide a reliable framework for data exchange.
Considerations when integrating blockchain include:
- Security: Provides enhanced protection against data breaches.
- Immutable Records: Changes to customer data can be tracked and verified, ensuring trustworthiness.
- Data Sharing: Facilitates seamless sharing of customer data between authorized entities without compromising security.
Adopting blockchain can fundamentally improve the efficiency of data operations while fostering a secure environment for data sharing among various stakeholders.
The future of customer data management is heavily influenced by innovative technologies such as AI, ML, and blockchain. Financial analysts must stay abreast of these trends to optimize their data strategies and enhance decision-making processes.
Ending
In this article, we have dissected the essential components involved in the creation of a customer database, particularly for financial analysts. The significance of this topic cannot be overstated. A well-structured customer database serves as the backbone for informed decision-making in the financial sector. It directly contributes to discerning customer behavior, preference patterns, and potential investment opportunities.
The following elements underscore the importance of creating a customer database:
- Tailored Insights: Financial analysts can harness data to create tailored financial products that align with individual customer needs, improving service delivery.
- Enhanced Strategy Formulation: A robust database aids in formulating investment strategies based on comprehensive customer profiles and historical data trends.
- Risk Management: Understanding customer data helps in identifying risks and managing them proactively.
Moreover, maintaining accuracy and compliance ensures the integrity of the data, which is critical in a highly regulated environment. Analysts must prioritize data quality and security throughout the database lifecycle.
The benefits are far-reaching; from generating actionable insights to fortifying customer relationships, the implications of an efficiently maintained customer database are profound. It is an element that every financial analyst should consider integral to their operations, especially in the modern landscape where data-driven decision making is paramount.
Summary of Key Points
To summarize, the steps discussed in the article include:
- Clearly defining data requirements before collection.
- Choosing the appropriate technology to manage and analyze this data.
- Implementing strong data privacy and compliance measures to protect customer information.
- Continuously auditing and updating the database to ensure its relevance and accuracy.
- Embracing advanced analytics to derive insights that guide strategic decisions.
These foundational steps contribute to the effectiveness of a customer database and enhance overall business operations.
Call to Action for Financial Analysts
Financial analysts are encouraged to take proactive steps in establishing a customer database. Here are some action points to guide your progress:
- Evaluate Current Practices: Review existing data collection and management processes to identify gaps.
- Invest in Appropriate Tools: Consider platforms like Microsoft SQL Server or Salesforce that align with your needs.
- Focus on Data Quality: Prioritize data accuracy through regular audits and updates.
- Stay Informed on Regulations: Keep abreast of privacy laws to ensure compliance and to build trust with your clients.
- Leverage Analytical Tools: Utilize tools like Tableau or Power BI to turn your data into actionable insights.
Building a comprehensive customer database is not simply an operational task but a strategic endeavor. By taking these steps, financial analysts can significantly enhance their ability to make informed, strategic investment decisions that resonate with the ever-evolving customer landscape.